Ten reasons to be cautious about using R to support decision-making during the COVID-19 pandemic
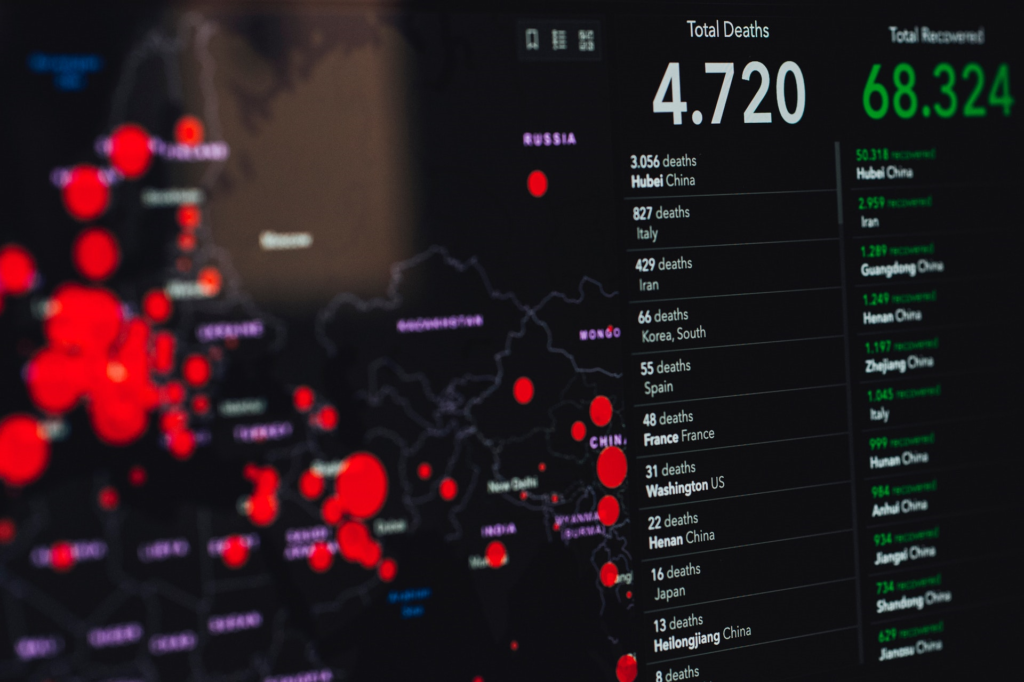
The risk is that a complicated number is released without context into a world that doesn’t know how to think about it.
The ‘R number’ that most of us non-epidemiologists had never heard of before appears to be driving government policies everywhere and determining how we lead our lives.
The UK Government has declared that R is currently running somewhere between 0.5 and 0.9 for the UK. Scotland’s R number is estimated to be between 0.7 and 1, while Wales has narrowed their R number down to around 0.8. The Northern Ireland First Minister has been even more precise, recently quoting a figure of 0.79, stating that it needed to be reduced further before restrictions can begin to be lifted.
R is defined as the average number of secondary infections produced by a single infectious individual. In the simplest of terms, if R is above 1 (i.e. an infectious person is, on average, passing the infection on to more than one other person) then the outbreak is expected to continue and to spread exponentially. If, however, it can be kept below 1 the outbreak will be kept under control and will eventually die out.
It is important to be aware, however, that the accuracy or otherwise of estimates of R will depend on the assumptions made, which may be erroneous, the quality of the data, which may be poor, and the epidemiological model used, of which there are many.
This article examines some of the caveats which apply to the use of R; it is a summary of a longer paper we have just published: When ‘R’ we going to get back to high fives, hugging strangers and kissing the Blarney Stone?
How useful is R?
R is a useful theoretical concept, as long as its limitations are understood and it is used alongside other available information.
It can be used, for example, in conjunction with other information, to forecast demand for hospital beds, demand for ICU beds, number of ventilators required, or likely number of deaths under different scenarios.
The basic reproduction number R0 provides a useful baseline and an indication of a disease’s potential. It can also be used to estimate the proportion of the population that would have to become immunised (either by acquiring the disease and recovering, or by vaccination) to attain herd immunity.
In its most basic form, R is essentially the product of three factors:
- The average rate of contact between susceptible and infected individuals;
- the probability of infection given contact between a susceptible and infected individual; and
- the duration of infectiousness.
However, very little of this information is easily obtainable. Even the duration of infectiousness can only be estimated within a range of values as there is some debate about when an individual becomes infectious before displaying symptoms. In the absence of extensive testing and contact tracing, R therefore has to be estimated indirectly from other sources, such as changes over time in new cases, hospital admissions, or deaths.
While R provides a useful indication of the potential of the disease, helps to inform policy in the fight against the disease, and gives some indication of what progress has been made in that fight, it is necessary to be aware of its limitations and weaknesses. The information going into the equations used to estimate R is far from perfect. Some of the problems that the scientists are faced with at the moment are as follows.
1. We do not know how many are infected
It is not known for sure how many people might be infected. The number of reported cases will depend on how many are being tested and who is being tested. A recent pilot survey carried out by the Office for National Statistics (ONS) produced an estimate of 0.27% of the population (excluding nursing homes, hospitals and other institutions). However, the confidence interval for this was stated to be between 0.17% and 0.41%, and this was based on 33 individuals testing positive, with some question marks over the number of false-positive and false-negative results. While there are plans to expand the survey and to include test results for antibodies, the figures are of limited use at present.
2. We do not know how many have immunity
Without reliable information on the number of people who have been infected, it is not possible to estimate the number in the population who may have acquired immunity and therefore the number who may still be susceptible. The antibody test part of the ONS infection survey may help to provide some information on that in the future, however.
3. The picture is changing as testing increases
The roll out of increased testing to more people and to different groups makes it difficult to identify an underlying trend in the number of infections. As the number of tests are increased, the likelihood of identifying new cases is also increased, so it is impossible to say how much of any change over time is due to the rate of spread of the virus. Also, as the people being tested do not provide a random sample, the number of cases cannot be used to estimate the number in the population who have been infected. So the figures for new cases or the cumulative number of cases are of little value, other than to give an indication of the least number of people infected.
4. Incubation periods vary
While the average incubation period is thought to be somewhere around 5 days (with a range of 2-14 days), different studies have come up with different estimates (see Worldometer on incubation). So this is another source of uncertainty. It is not possible therefore to trace the disease back to the time of infection, except through contact tracing.
5. Duration of infectiousness also varies
The duration of infectiousness appears to vary greatly, according to the severity of the disease. As pointed out earlier, estimates of the average duration of infectiousness also vary, with some uncertainty around how soon before displaying symptoms an individual might become infectious. Also, some people may be infectious without displaying any symptoms. These people may be less infectious than those with symptoms, but they are impossible to identify without testing.
6. There is a time lag in the reporting
The problem of delays in the reporting and registering of deaths has been well documented. Also, the different sets of figures produced from daily reports and from death registrations does not help. The average time period between infection and death added to delays in reporting means that information on deaths will always be 3 to 4 weeks behind the actual spread of infections (based on a Lancet article giving an estimate of around 18 days between the onset of symptoms and death, and adding an estimated average of 5 days for symptoms to develop, plus a couple of days for reporting delays). Uncertainty around the duration of the time lag, in itself, presents a problem for the models used.
7. Death rates change
Death rates can change as more vulnerable people die, leaving the disease with less vulnerable people to kill. Death rates can also change due to hospitals getting better at treating the disease. While changes in death rates can be mitigated to some extent by making different assumptions about different age groups or different ethnic groups, there are many other factors, such as obesity and various comorbidities, which have been linked to vulnerability.
8. Hospital admissions data do not provide a complete picture
Hospital admissions data provide a useful indication of trends in the spread of the disease, but do not include people being treated in care homes or those who may be seriously ill or dying at home. It also suffers from the same time lag problem as data on deaths, although with hospital admissions occurring around 3-6 days after the onset of symptoms (see this Patient.info article on disease timeline) the time lag would only be around 1 to 2 weeks.
9. R values are historical
The time lag between infection and the availability of data to input into the models means that any R values produced are, in fact, historical (i.e. at best, R reflects what was happening a few weeks previously). This limits the value of R for surveillance purposes, as it is out of date and a lot can change in the activity of the virus in a few weeks (see the recent Telegraph article by Sarah Knapton on this).
10. An amalgam of assumptions leads to a large range of error
Many assumptions have to be made in the various models used, such as the assumption that the entire population is susceptible at the outset of the disease, the assumption of immunity after recovery from the disease, or the assumption used by the Imperial College team that infected people with symptoms are 50% more infectious than infected people without symptoms. All these assumptions lead to a potentially large range of error in the numbers produced by the models.
Given the uncertainty that arises from all of this, R and many of the other numbers associated with COVID-19 must be interpreted with considerable caution. The many assumptions and estimates built into the models, the quality of the data, and the time lag in the data, could potentially lead to wrong decisions being taken at the wrong time, if too much reliance is placed on a single number.
Where ‘R’ we now?
If a vaccine can be found and the proportion of the population that have acquired immunity can be deduced (using antibody tests), R0 can be used to estimate the remaining proportion of the population that have to be vaccinated. In doing so, however, regional variations in R0 need to be taken into account as R0 varies with population urbanisation or ‘lived density’ (see Alasdair Rae’s article on this concept). Also, certain more vulnerable and high risk sub-groups (e.g. older people, vulnerable ethnic groups, health and social care workers) would have to be targeted in any vaccination programme.
While R does vary according to ‘lived density’ and other regional specific factors, the proliferation of regional Rs emerging does not help when it comes to policy making. The USA has a different R number for every state, with hugely varying confidence intervals attached to each of them (see rt.live). The UK and its devolved administrations have their own separate estimates of R, and different R numbers have lately appeared for different regions in England. The existence of regional Rs render the R at a national level fairly meaningless. Different councils in England have decided to be guided by their own regional circumstances when it comes to following government advice on, for example, returning to schools (see an FT article[1] on this).
Different regional administrations can now use their own R number to support their decision to take a different path from the one recommended at a national level. How far will this go? Do we need different Rs for different settings (e.g. care homes, hospitals) as well as different geographical areas? Within Northern Ireland, for example, do we need different Rs for different council areas? Should a Northern Ireland R be applied equally to Belfast and Belleek?
And where ‘R’ we heading?
In the meantime, it is necessary to continue indefinitely with what the Imperial College COVID-19 team refer to as ‘non-pharmaceutical interventions’ or, to use the more appropriate term, ‘suppression strategies’.
In their 25 January report, the Imperial College team concluded that control measures would need to block well over 60% of transmission to be effective in controlling the outbreak. It looks like that target has been achieved with the R number now less than 1 just about everywhere, albeit with a huge social and economic cost and untold collateral damage.
They also concluded (in their 16 March report) that such measures would have to be maintained until a vaccine becomes available (potentially 18 months or more), given the prediction that transmission will quickly rebound if interventions are relaxed. In addition, they suggested that a policy of intermittent social distancing (triggered by trends in disease surveillance) could be introduced. This would allow interventions to be relaxed temporarily in relative short time windows, but it would be necessary to quickly re-introduce control measures if or when case numbers began to rise again.
That is why it is necessary to continuously monitor R (or the growing number of Rs, along with other data on hospital admissions, ICU beds occupied, etc.) at least until the end of 2021. The results of this monitoring, along with observations of the unfolding events in other countries, will most likely determine the extent and nature of our social and economic activity for the next 18 months or so.
The scientists who are grinding out the R numbers are undoubtedly aware of all the caveats and weaknesses in their methods. Such awareness, however, appears to be lacking in the message presented to the public by government Ministers. In their attempts to keep the message simple and to convey a sense of being in control of the situation, they will no doubt be inclined to quote the latest R number, without confidence intervals and caveats, in support of their actions.
Increased testing and contact tracing will hopefully improve the estimates of R, assuming the UK and the devolved administrations can catch up in this area with other countries, such as Germany, South Korea and New Zealand (see a recent RaISe research paper on this).
If governments follow the R number slavishly, however, and the estimates are too high, the population will be paying a higher price than necessary in terms of social, economic and collateral damage. If the estimates of R are too low, there is the risk of easing restrictions too early, potentially giving rise to additional unnecessary deaths and prolonged misery. In the end, it all boils down to making the right judgement call and not relying too heavily on the numbers.
Ville Aula, a researcher at the London School of Economics, refers to the misplaced trust that the media and the public have in the COVID-19 numbers that are being rolled out every day, and concludes that:
Ultimately, the constant stream of empty numbers will grant us neither certainty nor solace.
Time will tell whether or not our trust in R is misplaced.
—————–
[1] Andy Bounds, Peter Foster & Sebastian Payne. 18 May 2020. ‘At least 300 English primary schools will not open to more pupils on June 1’, Financial Times.